250x250
Notice
Recent Posts
Recent Comments
Link
일 | 월 | 화 | 수 | 목 | 금 | 토 |
---|---|---|---|---|---|---|
1 | 2 | 3 | 4 | 5 | ||
6 | 7 | 8 | 9 | 10 | 11 | 12 |
13 | 14 | 15 | 16 | 17 | 18 | 19 |
20 | 21 | 22 | 23 | 24 | 25 | 26 |
27 | 28 | 29 | 30 |
Tags
- __len__
- items()
- node.js
- 파이썬
- CSS
- MySQL
- randrange()
- zipfile
- fnmatch
- glob
- __annotations__
- discard()
- JS
- mro()
- shuffle()
- locals()
- choice()
- __sub__
- count()
- decode()
- remove()
- inplace()
- MySqlDB
- __getitem__
- 오버라이딩
- Database
- View
- HTML
- shutil
- fileinput
Archives
- Today
- Total
흰둥이는 코드를 짤 때 짖어 (왈!왈!왈!왈!왈!왈!왈!왈!왈!왈!왈!)
(Python) 떡볶이집의 입점전략 본문
728x90
반응형
In [ ]:
import pandas as pd
In [ ]:
df = pd.read_csv('/content/drive/MyDrive/KDT/2. 데이터분석/소상공인시장진흥공단_상가(상권)정보_서울_202303.csv')
<ipython-input-85-597e531e1ed3>:1: DtypeWarning: Columns (2) have mixed types. Specify dtype option on import or set low_memory=False.
df = pd.read_csv('/content/drive/MyDrive/KDT/2. 데이터분석/소상공인시장진흥공단_상가(상권)정보_서울_202303.csv')
In [ ]:
df
Out[ ]:
상가업소번호 | 상호명 | 지점명 | 상권업종대분류코드 | 상권업종대분류명 | 상권업종중분류코드 | 상권업종중분류명 | 상권업종소분류코드 | 상권업종소분류명 | 표준산업분류코드 | ... | 건물관리번호 | 건물명 | 도로명주소 | 구우편번호 | 신우편번호 | 동정보 | 층정보 | 호정보 | 경도 | 위도 | |
---|---|---|---|---|---|---|---|---|---|---|---|---|---|---|---|---|---|---|---|---|---|
0 | MA0101202210A0037544 | 영자's곱창 | NaN | I2 | 음식 | I201 | 한식 | I20109 | 곱창 전골/구이 | I56111 | ... | 1121510100101690021003140 | NaN | 서울특별시 광진구 면목로 172 | 143900 | 4904 | NaN | 1 | NaN | 127.082832 | 37.568503 |
1 | MA0101202210A0097916 | 호텔클로이 | NaN | I1 | 숙박 | I101 | 일반 숙박 | I10102 | 여관/모텔 | I55102 | ... | 1114014500101300000014092 | 유성장 | 서울특별시 중구 을지로42길 11 | 100411 | 4561 | NaN | NaN | NaN | 127.005849 | 37.565708 |
2 | MA0101202210A0096343 | 나라차중계태권도장 | NaN | P1 | 교육 | P106 | 기타 교육 | P10601 | 태권도/무술학원 | P85611 | ... | 1135010600103640019010971 | 삼부프라자 | 서울특별시 노원구 한글비석로 242 | 139861 | 1734 | NaN | NaN | NaN | 127.076956 | 37.649226 |
3 | MA0101202210A0093845 | 이상한스냅 | NaN | M1 | 과학·기술 | M113 | 사진 촬영 | M11301 | 사진촬영업 | M73303 | ... | 1147010300100090004000001 | 백송주택 | 서울특별시 양천구 월정로 283 | 158822 | 7902 | NaN | NaN | NaN | 126.828832 | 37.542117 |
4 | MA010120220805430767 | 토끼정 | NaN | I2 | 음식 | I201 | 한식 | I20101 | 백반/한정식 | I56111 | ... | 1150010500107740012000001 | 마커스빌딩 | 서울특별시 강서구 마곡동로 55 | 157805 | 7802 | NaN | 2 | NaN | 126.833022 | 37.560053 |
... | ... | ... | ... | ... | ... | ... | ... | ... | ... | ... | ... | ... | ... | ... | ... | ... | ... | ... | ... | ... | ... |
474255 | MA0101202302A0049414 | 행복한부동산중개사무소 | NaN | L1 | 부동산 | L102 | 부동산 서비스 | L10203 | 부동산 중개/대리업 | L68221 | ... | 1147010300101670002011800 | NaN | 서울특별시 양천구 남부순환로 374 | 158829 | 7910 | NaN | NaN | NaN | 126.829372 | 37.533608 |
474256 | MA0101202302A0001316 | 좋은소식법률사무소 | NaN | M1 | 과학·기술 | M103 | 법무관련 | M10301 | 변호사 | M71101 | ... | 1121510700100200030025643 | NaN | 서울특별시 광진구 동일로30길 16 | 143916 | 5007 | NaN | 1 | NaN | 127.068072 | 37.547501 |
474257 | MA0101202302A0036033 | 루젤라 | NaN | I2 | 음식 | I204 | 서양식 | I20401 | 경양식 | I56123 | ... | 1168010800102110021000002 | 펜트힐루논현 | 서울특별시 강남구 언주로 641 | 135996 | 6105 | NaN | 1 | NaN | 127.034849 | 37.513922 |
474258 | MA0101202302A0049679 | 153커피 | NaN | S2 | 수리·개인 | S205 | 가전제품 수리 | S20501 | 가전제품 수리업 | S95310 | ... | 1156012800102380003019433 | NaN | 서울특별시 영등포구 양평로20길 14-2 | 150867 | 7206 | NaN | NaN | NaN | 126.896172 | 37.538297 |
474259 | MA0101202302A0109595 | 송죽상회 | NaN | G2 | 소매 | G205 | 식료품 소매 | G20504 | 건어물/젓갈 소매업 | G47213 | ... | 1114015100100760001012670 | NaN | 서울특별시 중구 을지로 230-1 | 100195 | 4561 | NaN | 3 | NaN | 127.003676 | 37.566546 |
474260 rows × 39 columns
In [ ]:
df.info()
<class 'pandas.core.frame.DataFrame'>
RangeIndex: 474260 entries, 0 to 474259
Data columns (total 39 columns):
# Column Non-Null Count Dtype
--- ------ -------------- -----
0 상가업소번호 474260 non-null object
1 상호명 474260 non-null object
2 지점명 1216 non-null object
3 상권업종대분류코드 474260 non-null object
4 상권업종대분류명 474260 non-null object
5 상권업종중분류코드 474260 non-null object
6 상권업종중분류명 474260 non-null object
7 상권업종소분류코드 474260 non-null object
8 상권업종소분류명 474260 non-null object
9 표준산업분류코드 474260 non-null object
10 표준산업분류명 474260 non-null object
11 시도코드 474260 non-null int64
12 시도명 474260 non-null object
13 시군구코드 474260 non-null int64
14 시군구명 474260 non-null object
15 행정동코드 474260 non-null int64
16 행정동명 474260 non-null object
17 법정동코드 474260 non-null int64
18 법정동명 474260 non-null object
19 지번코드 474260 non-null int64
20 대지구분코드 474260 non-null int64
21 대지구분명 474260 non-null object
22 지번본번지 474260 non-null int64
23 지번부번지 395801 non-null float64
24 지번주소 474260 non-null object
25 도로명코드 474260 non-null int64
26 도로명 474260 non-null object
27 건물본번지 474259 non-null float64
28 건물부번지 62306 non-null float64
29 건물관리번호 474256 non-null object
30 건물명 223494 non-null object
31 도로명주소 474260 non-null object
32 구우편번호 474260 non-null int64
33 신우편번호 474260 non-null int64
34 동정보 0 non-null float64
35 층정보 326900 non-null object
36 호정보 0 non-null float64
37 경도 474260 non-null float64
38 위도 474260 non-null float64
dtypes: float64(7), int64(10), object(22)
memory usage: 141.1+ MB
In [ ]:
shop = ['엽기떡볶이', '신전떡볶이', '죠스떡볶이', '국대떡볶이', '감탄떡볶이']
In [ ]:
# 파리바게트(파리바게뜨) 데이터 필터링하기
# df_paris
# '상가업소번호', '상호명, '경도', '위도'
# contains(): 특정 문자열 포함여부를 True, False로 반환
cont1 = df['상호명'].str.contains(r'파리바게뜨|파리바게트')
df_paris = df.loc[cont1, ['상가업소번호', '상호명', '경도', '위도']].copy()
df_paris
# df.loc[df['상호명'] == '파리바게뜨', ['상가업소번호', '상호명', '경도', '위도']]
Out[ ]:
상가업소번호 | 상호명 | 경도 | 위도 | |
---|---|---|---|---|
1225 | MA010120220805464775 | 파리바게뜨풍납초교점 | 127.117232 | 37.534101 |
2603 | MA010120220805503393 | 파리바게뜨 | 127.086178 | 37.594830 |
3016 | MA010120220805515071 | 파리바게뜨오금현대 | 127.128655 | 37.507317 |
6824 | MA010120220805839680 | 파리바게트그라시움 | 127.159471 | 37.556197 |
7749 | MA010120220805926470 | 파리바게트양재역 | 127.034934 | 37.482755 |
... | ... | ... | ... | ... |
467693 | MA0101202301A0046528 | 파리바게뜨서초우면점 | 127.024937 | 37.470698 |
469312 | MA0101202302A0012597 | 파리바게뜨문정 | 127.129498 | 37.483436 |
470560 | MA0101202302A0083824 | 파리바게뜨문정카페점 | 127.131290 | 37.487792 |
472672 | MA0101202302A0059905 | 파리바게뜨레이크펠리스점 | 127.092487 | 37.507008 |
474144 | MA0101202302A0059526 | 파리바게뜨상계 | 127.074410 | 37.658100 |
598 rows × 4 columns
In [ ]:
df_paris = df_paris.set_axis(['업소번호', '업소상호명', '업소경도', '업소위도'], axis=1).reset_index(drop=True)
df_paris
Out[ ]:
업소번호 | 업소상호명 | 업소경도 | 업소위도 | |
---|---|---|---|---|
0 | MA010120220805464775 | 파리바게뜨풍납초교점 | 127.117232 | 37.534101 |
1 | MA010120220805503393 | 파리바게뜨 | 127.086178 | 37.594830 |
2 | MA010120220805515071 | 파리바게뜨오금현대 | 127.128655 | 37.507317 |
3 | MA010120220805839680 | 파리바게트그라시움 | 127.159471 | 37.556197 |
4 | MA010120220805926470 | 파리바게트양재역 | 127.034934 | 37.482755 |
... | ... | ... | ... | ... |
593 | MA0101202301A0046528 | 파리바게뜨서초우면점 | 127.024937 | 37.470698 |
594 | MA0101202302A0012597 | 파리바게뜨문정 | 127.129498 | 37.483436 |
595 | MA0101202302A0083824 | 파리바게뜨문정카페점 | 127.131290 | 37.487792 |
596 | MA0101202302A0059905 | 파리바게뜨레이크펠리스점 | 127.092487 | 37.507008 |
597 | MA0101202302A0059526 | 파리바게뜨상계 | 127.074410 | 37.658100 |
598 rows × 4 columns
In [ ]:
# 떡볶이 프랜차이즈 데이터 필터링
# extract(): 특정 문자열을 포함하고 있다면 그 문자열을 반환하고, 포함하고 있지 않다면 NaN을 반환
df_shop = df.copy()
df_shop['상호명'] = df_shop['상호명'].str.extract(r'({})'.format('|'.join(shop)))[0]
df_shop = df_shop.dropna(subset=['상호명']).iloc[:, [0, 1, 14, 37, 38]].reset_index(drop=True)
df_shop
Out[ ]:
상가업소번호 | 상호명 | 시군구명 | 경도 | 위도 | |
---|---|---|---|---|---|
0 | MA010120220805508370 | 엽기떡볶이 | 광진구 | 127.083078 | 37.537400 |
1 | MA010120220805541204 | 엽기떡볶이 | 은평구 | 126.919784 | 37.618410 |
2 | MA010120220805693796 | 엽기떡볶이 | 관악구 | 126.913418 | 37.485890 |
3 | MA010120220805725890 | 엽기떡볶이 | 노원구 | 127.075399 | 37.658792 |
4 | MA010120220805729207 | 신전떡볶이 | 강동구 | 127.155062 | 37.552742 |
... | ... | ... | ... | ... | ... |
295 | MA0101202212A0089517 | 신전떡볶이 | 용산구 | 126.969033 | 37.544686 |
296 | MA0101202301A0075280 | 신전떡볶이 | 동대문구 | 127.051415 | 37.591141 |
297 | MA0101202302A0019465 | 엽기떡볶이 | 성북구 | 127.011724 | 37.605061 |
298 | MA0101202302A0032718 | 엽기떡볶이 | 강동구 | 127.146476 | 37.550391 |
299 | MA0101202301A0037432 | 감탄떡볶이 | 노원구 | 127.061300 | 37.649715 |
300 rows × 5 columns
In [ ]:
# 곱집합
df1 = pd.DataFrame(['A', 'B'])
df2 = pd.DataFrame(['가', '나', '다'])
df1.merge(df2, how='cross')
Out[ ]:
0_x | 0_y | |
---|---|---|
0 | A | 가 |
1 | A | 나 |
2 | A | 다 |
3 | B | 가 |
4 | B | 나 |
5 | B | 다 |
In [ ]:
df_cross = df_shop.merge(df_paris, how='cross')
df_cross
Out[ ]:
상가업소번호 | 상호명 | 시군구명 | 경도 | 위도 | 업소번호 | 업소상호명 | 업소경도 | 업소위도 | |
---|---|---|---|---|---|---|---|---|---|
0 | MA010120220805508370 | 엽기떡볶이 | 광진구 | 127.083078 | 37.537400 | MA010120220805464775 | 파리바게뜨풍납초교점 | 127.117232 | 37.534101 |
1 | MA010120220805508370 | 엽기떡볶이 | 광진구 | 127.083078 | 37.537400 | MA010120220805503393 | 파리바게뜨 | 127.086178 | 37.594830 |
2 | MA010120220805508370 | 엽기떡볶이 | 광진구 | 127.083078 | 37.537400 | MA010120220805515071 | 파리바게뜨오금현대 | 127.128655 | 37.507317 |
3 | MA010120220805508370 | 엽기떡볶이 | 광진구 | 127.083078 | 37.537400 | MA010120220805839680 | 파리바게트그라시움 | 127.159471 | 37.556197 |
4 | MA010120220805508370 | 엽기떡볶이 | 광진구 | 127.083078 | 37.537400 | MA010120220805926470 | 파리바게트양재역 | 127.034934 | 37.482755 |
... | ... | ... | ... | ... | ... | ... | ... | ... | ... |
179395 | MA0101202301A0037432 | 감탄떡볶이 | 노원구 | 127.061300 | 37.649715 | MA0101202301A0046528 | 파리바게뜨서초우면점 | 127.024937 | 37.470698 |
179396 | MA0101202301A0037432 | 감탄떡볶이 | 노원구 | 127.061300 | 37.649715 | MA0101202302A0012597 | 파리바게뜨문정 | 127.129498 | 37.483436 |
179397 | MA0101202301A0037432 | 감탄떡볶이 | 노원구 | 127.061300 | 37.649715 | MA0101202302A0083824 | 파리바게뜨문정카페점 | 127.131290 | 37.487792 |
179398 | MA0101202301A0037432 | 감탄떡볶이 | 노원구 | 127.061300 | 37.649715 | MA0101202302A0059905 | 파리바게뜨레이크펠리스점 | 127.092487 | 37.507008 |
179399 | MA0101202301A0037432 | 감탄떡볶이 | 노원구 | 127.061300 | 37.649715 | MA0101202302A0059526 | 파리바게뜨상계 | 127.074410 | 37.658100 |
179400 rows × 9 columns
In [ ]:
# 두 지점의 위도와 경도를 입력하면 거리를 구해주는 라이브러리
!pip install haversine
Looking in indexes: https://pypi.org/simple, https://us-python.pkg.dev/colab-wheels/public/simple/
Requirement already satisfied: haversine in /usr/local/lib/python3.10/dist-packages (2.8.0)
In [ ]:
from haversine import haversine
In [ ]:
seoul = [37.541, 126.986]
paris = [48.8567, 2.3508]
haversine(seoul, paris, unit='m')
Out[ ]:
8968562.580161477
In [ ]:
df_cross['거리'] = df_cross.apply(lambda x: haversine([x[4], x[3]], [x[8], x[7]], unit='m'), axis=1)
df_cross
Out[ ]:
상가업소번호 | 상호명 | 시군구명 | 경도 | 위도 | 업소번호 | 업소상호명 | 업소경도 | 업소위도 | 거리 | |
---|---|---|---|---|---|---|---|---|---|---|
0 | MA010120220805508370 | 엽기떡볶이 | 광진구 | 127.083078 | 37.537400 | MA010120220805464775 | 파리바게뜨풍납초교점 | 127.117232 | 37.534101 | 3033.819143 |
1 | MA010120220805508370 | 엽기떡볶이 | 광진구 | 127.083078 | 37.537400 | MA010120220805503393 | 파리바게뜨 | 127.086178 | 37.594830 | 6391.763178 |
2 | MA010120220805508370 | 엽기떡볶이 | 광진구 | 127.083078 | 37.537400 | MA010120220805515071 | 파리바게뜨오금현대 | 127.128655 | 37.507317 | 5229.360832 |
3 | MA010120220805508370 | 엽기떡볶이 | 광진구 | 127.083078 | 37.537400 | MA010120220805839680 | 파리바게트그라시움 | 127.159471 | 37.556197 | 7051.848831 |
4 | MA010120220805508370 | 엽기떡볶이 | 광진구 | 127.083078 | 37.537400 | MA010120220805926470 | 파리바게트양재역 | 127.034934 | 37.482755 | 7413.113137 |
... | ... | ... | ... | ... | ... | ... | ... | ... | ... | ... |
179395 | MA0101202301A0037432 | 감탄떡볶이 | 노원구 | 127.061300 | 37.649715 | MA0101202301A0046528 | 파리바게뜨서초우면점 | 127.024937 | 37.470698 | 20162.267554 |
179396 | MA0101202301A0037432 | 감탄떡볶이 | 노원구 | 127.061300 | 37.649715 | MA0101202302A0012597 | 파리바게뜨문정 | 127.129498 | 37.483436 | 19441.977917 |
179397 | MA0101202301A0037432 | 감탄떡볶이 | 노원구 | 127.061300 | 37.649715 | MA0101202302A0083824 | 파리바게뜨문정카페점 | 127.131290 | 37.487792 | 19032.461961 |
179398 | MA0101202301A0037432 | 감탄떡볶이 | 노원구 | 127.061300 | 37.649715 | MA0101202302A0059905 | 파리바게뜨레이크펠리스점 | 127.092487 | 37.507008 | 16104.564315 |
179399 | MA0101202301A0037432 | 감탄떡볶이 | 노원구 | 127.061300 | 37.649715 | MA0101202302A0059526 | 파리바게뜨상계 | 127.074410 | 37.658100 | 1483.587708 |
179400 rows × 10 columns
In [ ]:
# 개별 떡볶이 매장과 파리바게트와의 거리
df_dis = df_cross.groupby(['상가업소번호', '상호명'])['거리'].min().reset_index()
df_dis
Out[ ]:
상가업소번호 | 상호명 | 거리 | |
---|---|---|---|
0 | MA010120220800007341 | 엽기떡볶이 | 45.677208 |
1 | MA010120220800033857 | 죠스떡볶이 | 323.845827 |
2 | MA010120220800040224 | 엽기떡볶이 | 348.538405 |
3 | MA010120220800106006 | 신전떡볶이 | 308.577801 |
4 | MA010120220800202270 | 엽기떡볶이 | 512.715119 |
... | ... | ... | ... |
295 | MA0101202301A0069471 | 엽기떡볶이 | 231.782042 |
296 | MA0101202301A0075280 | 신전떡볶이 | 168.269281 |
297 | MA0101202302A0003449 | 신전떡볶이 | 180.934977 |
298 | MA0101202302A0019465 | 엽기떡볶이 | 390.205288 |
299 | MA0101202302A0032718 | 엽기떡볶이 | 236.454718 |
300 rows × 3 columns
In [ ]:
# 각 프렌차이즈 별 파리바게트와의 평균 거리
df_dis.groupby('상호명')['거리'].mean()
Out[ ]:
상호명
감탄떡볶이 332.304595
국대떡볶이 144.372956
신전떡볶이 241.867223
엽기떡볶이 231.986667
죠스떡볶이 203.369123
Name: 거리, dtype: float64
In [ ]:
# agg(): 다중집계작업을 간결하게 해주는 함수
df_dis.groupby('상호명')['거리'].agg(['mean', 'count'])
Out[ ]:
mean | count | |
---|---|---|
상호명 | ||
감탄떡볶이 | 332.304595 | 12 |
국대떡볶이 | 144.372956 | 9 |
신전떡볶이 | 241.867223 | 88 |
엽기떡볶이 | 231.986667 | 129 |
죠스떡볶이 | 203.369123 | 62 |
In [ ]:
# 프랜차이즈 별 파리바게트와 50m이하인 매장의 평균거리와 매장개수
dis = df_dis['거리'] <= 50
df_dis[dis].groupby('상호명')['거리'].agg(['mean', 'count'])
Out[ ]:
mean | count | |
---|---|---|
상호명 | ||
감탄떡볶이 | 0.000000 | 1 |
국대떡볶이 | 8.697679 | 3 |
신전떡볶이 | 32.342522 | 4 |
엽기떡볶이 | 20.017968 | 9 |
죠스떡볶이 | 17.309602 | 13 |
In [ ]:
# 거리를 입력하면 프랜차이즈 별 파리바데트와의 평균거리와 매장개수를 출력하는 함수
# distance(x)
def distance(x):
dis = df_dis['거리'] <= x
return df_dis[dis].groupby('상호명')['거리'].agg(['mean', 'count'])
distance(50)
Out[ ]:
mean | count | |
---|---|---|
상호명 | ||
감탄떡볶이 | 0.000000 | 1 |
국대떡볶이 | 8.697679 | 3 |
신전떡볶이 | 32.342522 | 4 |
엽기떡볶이 | 20.017968 | 9 |
죠스떡볶이 | 17.309602 | 13 |
In [ ]:
!pip install pandasecharts
Looking in indexes: https://pypi.org/simple, https://us-python.pkg.dev/colab-wheels/public/simple/
Requirement already satisfied: pandasecharts in /usr/local/lib/python3.10/dist-packages (0.5)
Requirement already satisfied: pandas in /usr/local/lib/python3.10/dist-packages (from pandasecharts) (1.5.3)
Requirement already satisfied: pyecharts>=1.0.0 in /usr/local/lib/python3.10/dist-packages (from pandasecharts) (2.0.3)
Requirement already satisfied: jinja2 in /usr/local/lib/python3.10/dist-packages (from pyecharts>=1.0.0->pandasecharts) (3.1.2)
Requirement already satisfied: prettytable in /usr/local/lib/python3.10/dist-packages (from pyecharts>=1.0.0->pandasecharts) (0.7.2)
Requirement already satisfied: simplejson in /usr/local/lib/python3.10/dist-packages (from pyecharts>=1.0.0->pandasecharts) (3.19.1)
Requirement already satisfied: python-dateutil>=2.8.1 in /usr/local/lib/python3.10/dist-packages (from pandas->pandasecharts) (2.8.2)
Requirement already satisfied: pytz>=2020.1 in /usr/local/lib/python3.10/dist-packages (from pandas->pandasecharts) (2022.7.1)
Requirement already satisfied: numpy>=1.21.0 in /usr/local/lib/python3.10/dist-packages (from pandas->pandasecharts) (1.22.4)
Requirement already satisfied: six>=1.5 in /usr/local/lib/python3.10/dist-packages (from python-dateutil>=2.8.1->pandas->pandasecharts) (1.16.0)
Requirement already satisfied: MarkupSafe>=2.0 in /usr/local/lib/python3.10/dist-packages (from jinja2->pyecharts>=1.0.0->pandasecharts) (2.1.2)
In [ ]:
df_50 = distance(50).reset_index()
In [ ]:
df_50
Out[ ]:
상호명 | mean | count | |
---|---|---|---|
0 | 감탄떡볶이 | 0.000000 | 1 |
1 | 국대떡볶이 | 8.697679 | 3 |
2 | 신전떡볶이 | 32.342522 | 4 |
3 | 엽기떡볶이 | 20.017968 | 9 |
4 | 죠스떡볶이 | 17.309602 | 13 |
In [ ]:
from pandasecharts import echart
import IPython
In [ ]:
df_50.echart.pie(x='상호명', y='count', figsize=(600, 400),
radius=['20%', '60%'], label_opts={'position':'outer'},
title='떡볶이집의 입점 전략은 과연 파리바게트 옆인가?',
legend_opts={'pos_right':'0%', 'orient':'vertical'},
subtitle='50m 이내 매장수').render()
IPython.display.HTML(filename='render.html')
Out[ ]: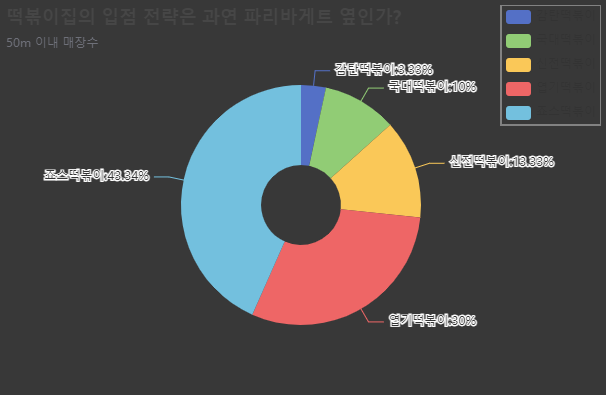
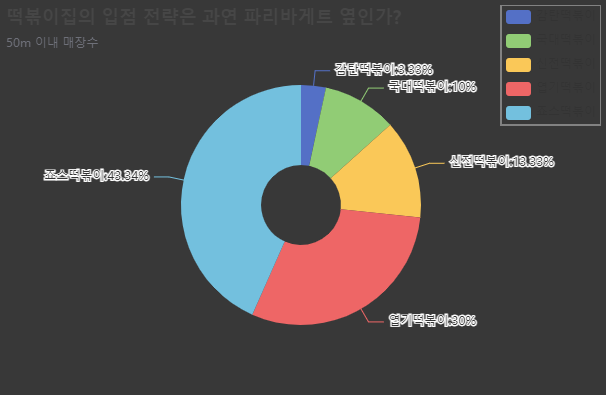
In [ ]:
from pyecharts.charts import Timeline, Grid
In [ ]:
tl = Timeline({'width':'600px', 'height':'400px'})
pie1 = df_50.echart.pie(x='상호명', y='count', figsize=(600, 400),
radius=['20%', '60%'], label_opts={'position':'outer'},
title='떡볶이집의 입점 전략은 과연 파리바게트 옆인가?',
legend_opts={'pos_right':'0%', 'orient':'vertical'},
subtitle='50m 이내 매장수')
tl.add(pie1, '50m').render()
IPython.display.HTML(filename='render.html')
Out[ ]: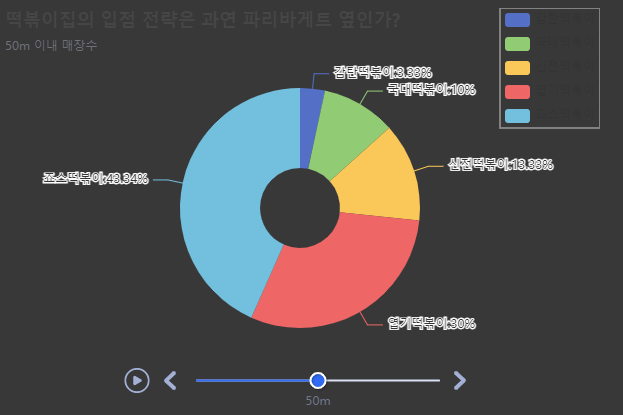
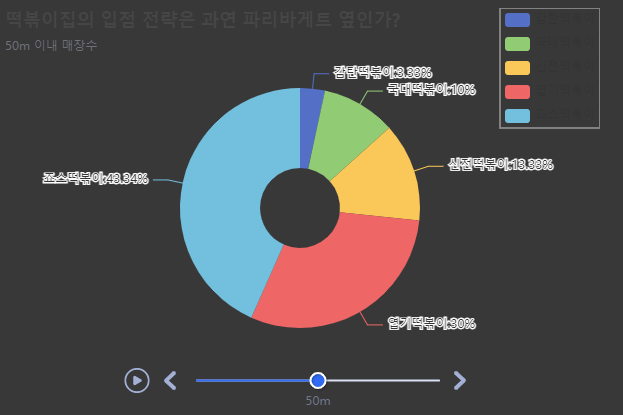
In [ ]:
tl = Timeline({'width':'600px', 'height':'400px'})
for i in [1000, 100, 50, 30]:
df_d = distance(i).reset_index()
pie1 = df_d.echart.pie(x='상호명', y='count', figsize=(600, 400),
radius=['20%', '60%'], label_opts={'position':'outer'},
title='떡볶이집의 입점 전략은 과연 파리바게트 옆인가?',
legend_opts={'pos_right':'0%', 'orient':'vertical'},
subtitle=f'{i}m 이내 매장수')
tl.add(pie1, f'{i}m').render()
IPython.display.HTML(filename='render.html')
Out[ ]: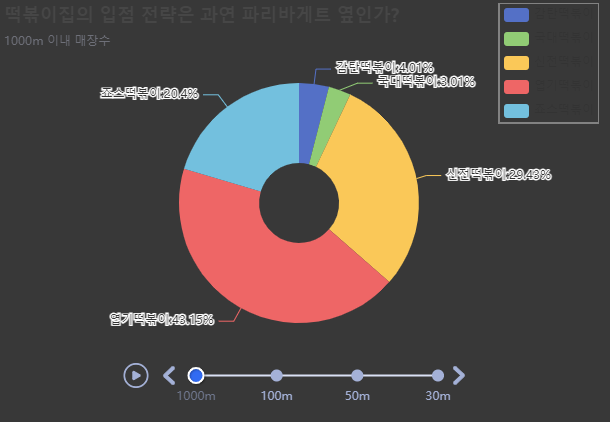
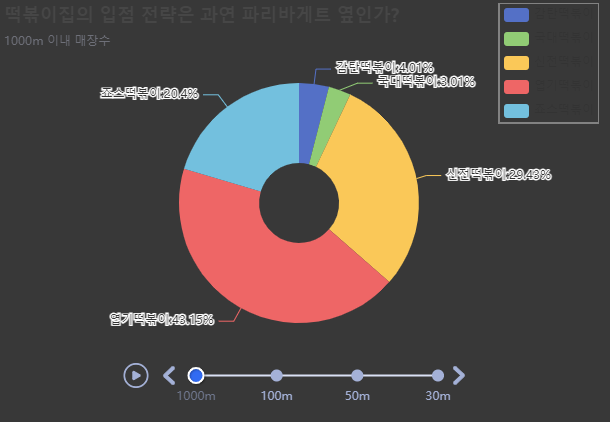
728x90
반응형
'파이썬 데이터 분석' 카테고리의 다른 글
(Python) 스타벅스 API를 이용한 프로젝트 (0) | 2023.06.09 |
---|---|
(Python) 따릉이 API를 이용한 프로젝트 (0) | 2023.06.09 |
(Python) 전국 도시공원 데이터 프로젝트 (0) | 2023.06.09 |
(Python) 상권별 업종 밀집 통계 프로젝트 (0) | 2023.06.09 |
(Python) folium (0) | 2023.06.08 |
Comments